
The subsequent linear association step has been widely applied in the statistical analyses of bulk RNA-seq and micro-array co-expression 17, genome-wide association studies 18, 19, expression quantitative trait loci 20, and causal network inference 21, 22. The normalization step (e.g., sctransform 14, bayNorm 15, and Sanity 16) removes confounding technical noises from raw read counts to recover the biological variations. These challenges limit the accuracy, efficiency, scalability, and flexibility of single-cell data analysis and the downstream biological discovery.Ī potential unified framework for single-cell DE, co-expression and CRISPR analysis is a two-step normalization–association inferential process (Fig. Single-cell CRISPR screen analysis can be regarded as multi-variate DE, but existing methods (e.g., scMageck 12 and SCEPTRE 13) take weeks or more on a modern dataset and do not account for off-target effects. 1, 9) are difficult to generalize to single-cell gene co-expression, leaving it susceptible to expression-dependent normalization biases 10, 11. A comprehensive benchmarking found that single-cell-specific attempts in DE could not outperform existing bulk methods such as edgeR 8. However, cell-to-cell technical variations and low read counts in scRNA-seq remain challenging in computational and statistical perspectives. Regarding and manipulating each cell independently, especially in combination with CRISPR (clustered regularly interspaced short palindromic repeats) technology 6, 7, scRNA-seq can overcome the major limitations in sample and perturbation richness of bulk studies, at a fraction of the cost. Single-cell RNA-seq (scRNA-seq) provides a unique glance into cellular transcriptomic variations beyond the capabilities of bulk technologies, enabling analyses such as single-cell differential expression (DE) 1, co-expression 2, 3, and causal network inference 4, 5 on cell subsets at will. RNA sequencing (RNA-seq) has received particular popularity for systematically screening transcriptional gene regulation and co-regulation. Understanding gene regulatory networks and their phenotypic outcomes forms a major part of biological studies. On conventional scRNA-seq, Normalisr recovers gene-level co-expression networks that recapitulated known gene functions. The superior scalability allows us to reconstruct robust gene regulatory networks from trans-effects of guide RNAs in large-scale single cell CRISPRi screens. By systematically detecting and removing nonlinear confounders arising from library size at mean and variance levels, Normalisr achieves high sensitivity, specificity, speed, and generalizability across multiple scRNA-seq protocols and experimental conditions with unbiased p-value estimation.
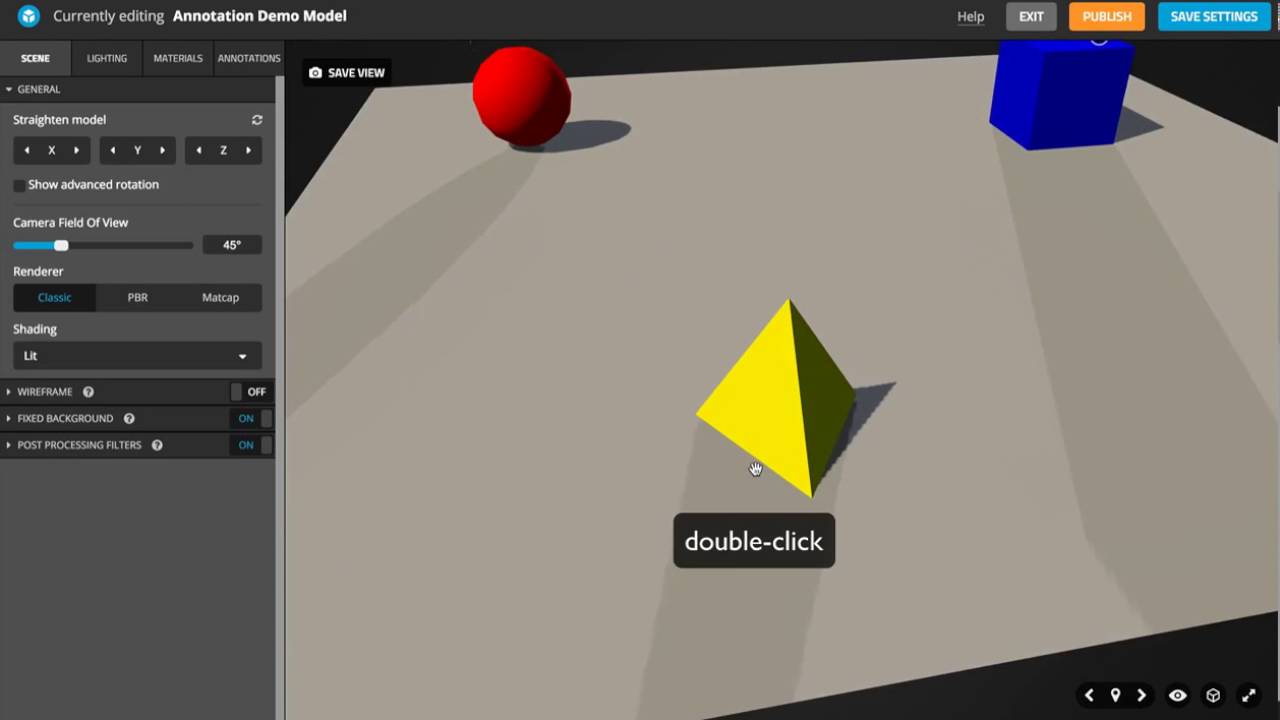
Here we present Normalisr, a normalization and statistical association testing framework that unifies single-cell differential expression, co-expression, and CRISPR screen analyses with linear models.

Furthermore, statistical association testing remains difficult for scRNA-seq. Single-cell RNA sequencing (scRNA-seq) provides unprecedented technical and statistical potential to study gene regulation but is subject to technical variations and sparsity.
